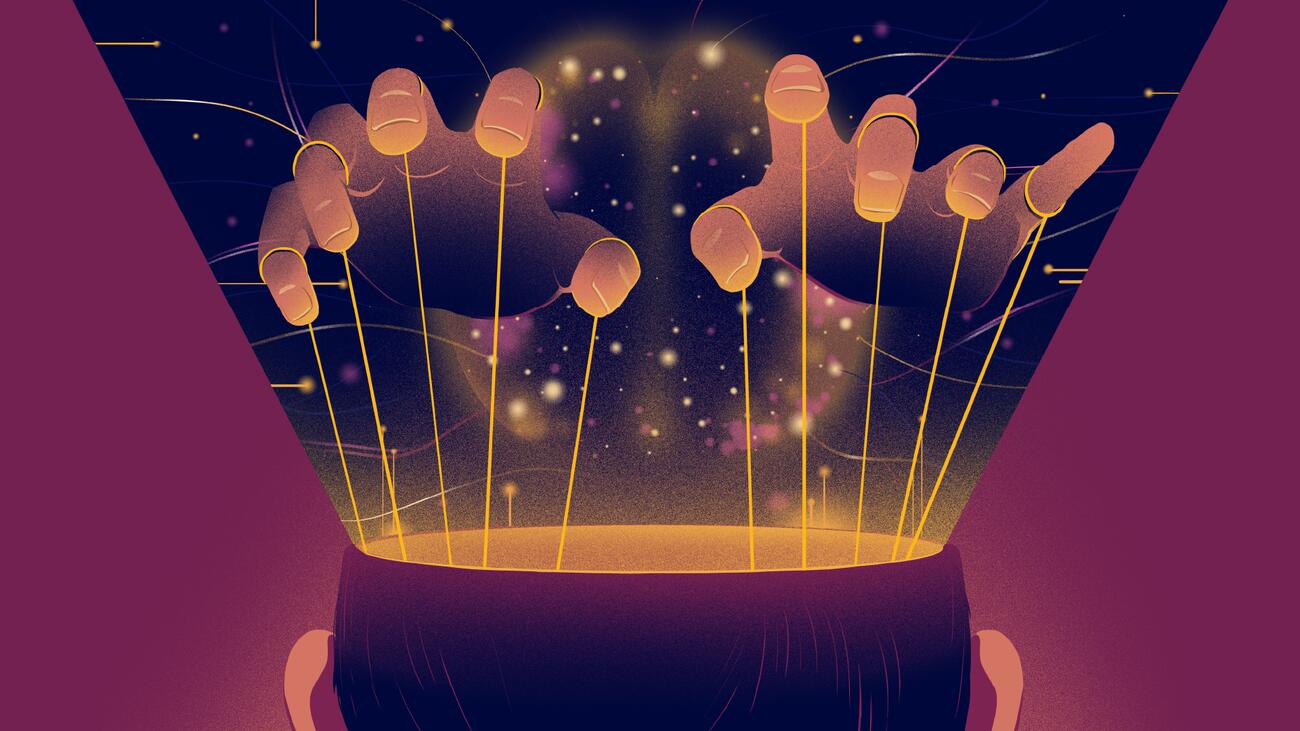
Illustration by Eric Chow
Code of ethics
How do we make artificial intelligence accountable to the people who use it?
“May you live in interesting times” – one of the most deliciously back-handed of English blessings.
Interesting times smacks of adventure and fulfillment, inferring the imagined prospect of uninteresting times – a curse upon a century of bored dullards who would give anything for a new colour or a self-driving horse.
But interesting times are usually periods of great disruption. Disruption means change, and change is scary. Three of the most disruptive creations in human history – the computer chip, the internet, and AI – have emerged in just the past 70 years. Only two generations separate us from a markedly primitive state of being.
It’s the nature of disruptive technology to obscure the future. We don’t know what disruption will bring, and these three latest have compounded one atop the next at such blinding speed that they’ve fundamentally changed an individual’s place in society from one of personal connections to one of data points – data that is being used, ironically, to teach artificial intelligence how to be more human.
The sudden ubiquity of AI has brought us to a precipice we are ill-equipped to understand, much less navigate. While the imagined threat of a genocidal machine sentience has been dominating the imagination (and the headlines), we’ve allowed ourselves to be overtaken by an actual – and far more insidious – threat: that AI was created mostly in secret, mostly for profit, and mostly from data we didn’t realize we were feeding it.
The results have led us into a hornet’s nest of issues with which we now have to contend – biased data, privacy infringement, subtle pushes into online social connections based on mathematical probabilities and digitally ingrained motives even our experts don’t fully grasp. No doubt the potential benefits of AI are almost limitless – it might produce a cure for cancer, an end to hunger and war, a previously unimagined pathway to justice and progress on a global scale.
But in the here and now, we have a very serious problem and not a lot of solutions. Is it possible to wrestle control from the data that shapes our lives behind our backs, to develop an ethical, equitable AI that will serve us more and milk us less? How can we ensure that AI will fulfill its potential to benefit all members of society – to be accurate, fair, and safe? How do we create a responsible future when we barely understand the technology that is taking us there?
“AI and data are human rights issues,” says Wendy H. Wong, professor of political science at UBC Okanagan, and author of We the Data: Human Rights in the Digital Age. “Human rights were conceived in a purely analog world. It’s no surprise we don't tend to think about data and the AI systems that use it as part of human rights. Until very recently data has not been about human activities at a very granular level. But that granular data that we collect today is changing how we understand people, how we understand ourselves, and how we understand how we fit into communities – this data tracks nearly every moment of our lives.”
MO’ DATA, MO’ PROBLEMS
To understand the scope of the problem, we have to break AI down into its three essential elements: the physical computing resources, the algorithm that runs on those resources, and the data that the algorithm plows through to find patterns and make predictions.
As robust as these elements are in concert, their individual limitations feed into a loop that amplifies biases and creates the potential for human-rights violations: the volume of the physical computing resources limits the size and complexity of the algorithm, which draws its information from a biased dataset that purports to be the sum of human experience.
Even if computing capacity continues to grow – and there’s no reason to think it wouldn’t – AI algorithms developed by private companies for competitive gain using biased data foster an understandable level of mistrust in their objectivity.
In a sense, we have gone from choosing our communities to having our communities chosen for us. Decisions we used to make actively – what voices to listen to, what communities to join – are now pushed upon us passively by an algorithm that makes decisions based on the laws of probability and the goals of commerce, threatening a social fabric that has thrived for thousands of years on personal relationships, cultural exchange, and good old-fashioned happenstance.
“A lot of algorithmic sorting is happening without our knowledge or even consent,” says Wong. “So the types of online communities we belong to have become very disconnected from our everyday lives.”
Equally disturbing, and perhaps more intractable, is the issue of personal data. We have not solved the thorny issue of who owns whose data once it is collected. And now, with decades of data in the bank, and our every move tracked, tabulated, and regurgitated to us in purchasable form, any real sense of privacy is a childhood memory.
“This is where we really run into some social problems,” says Wong. “One thing we have to come to terms with in how we think about the relationship between data and human societies is that, even as a data source, I can't fully claim that data is mine. It’s about me, but it’s not mine because it didn’t exist without some data collector or some company wanting to make data about a certain type of activity or a certain type of choice.”
Is it possible to wrestle control from the data that shapes our lives behind our backs, to develop an ethical, equitable AI that will serve us more and milk us less?
Beyond the algorithm, AI is only as good as the data that underlies it, and with most of that data collected for commercial purpose to appeal to certain types of people, the datasets are inherently biased, and certain types of information – including English language source material and Western cultural perspectives – are being privileged over others. So if you ask your chatbot to show you a picture of breakfast, you’re far more likely to see bacon and eggs than fried noodles and rice porridge.
“We each have our own cultural background that affects the way that we communicate and the way that we see the world and interpret each other,” says Vered Shwartz, an assistant professor of computer science who researches natural language processing (NLP). “And so we would like language models to be able to interact with people in their native language, to be able to understand them.”
Although experts have been working on NLP for multiple languages for many years, and large language models (LLMs) do exist for languages other than English, those that have been developed for low-resource languages (meaning there is simply less data available on which to train them) tend to be of lower quality. “These models are built with English in mind, and then applied to other languages, but there are properties in other languages – like morphology – that aren’t the same as English,” says Shwartz, who holds the Canada CIFAR AI chair at the Vector Institute. “The solutions, right now, are not great.” Even if an LLM is available in their native language, she says, people may choose to interact with English models instead.
Because of this, Shwartz’s research group is seeking ways to make English LLMs more culturally inclusive – one meal at a time. Currently they are collecting a dataset with images from 60 cultures to expand AI’s understanding of, among many other things, breakfasts that wouldn’t show up on an IHOP menu.
Shwartz also studies the potential for AI to grasp interactional data that lies beyond language – such as reading facial expressions, gestures, and tone of voice. The intent is to create a language model that not only understands languages, but grasps subtle differences in meaning and cultural significance. AI should be able to discern that a henna tattoo represents something different in an Indian wedding than it does at a fashion show, and that generative images of Nazi soldiers shouldn’t include Black troops out of a sense of inclusiveness (these are both recent, actual examples of AI figuring itself out).
In other words, responsible AI needs a dash of common sense – to be able to reason like human beings do. But even in this, there is contradiction. Humans reason very differently across cultures, and we all, from time to time, act well beyond any sense of reason. So how can we expect an artificial intelligence to learn reason from a species that has no common definition of the word, and doesn’t apply it with any consistency? And once AI figures it out, what impact will that have on society?
THE FINE ART OF THINKING FOR YOURSELF
“The way policymakers focus on AI and regulation is really unspecific and perhaps not very helpful,” says Wong. “We're focusing on the technological change without really playing out the underlying social, political, economic, and cultural changes that are happening.”
Policymakers aren’t generally known for being up on the latest tech, but with incredibly complex and rapidly growing technology, like the large language models that power AI, it’s understandable. Before LLMs, the advances in AI – particularly at the consumer level – were incremental and largely invisible, rolled out through customer support chatbots, social media algorithms, and virtual assistants like Siri and Alexa. But since OpenAI released ChatGPT-3.5 in March 2022, basically providing a free personal assistant to anyone with an internet connection, we have been using a tool that’s as mysterious and unreliable as it is impressive.
The understanding that policymakers have of computers will always fall short of the speed at which the tech evolves. Even academics who study AI have learned that their research is essentially out of date by the time it’s published. So in place of a comprehensive grok of the technology, there is an immediate need to make the public “data literate” – not teaching the technical aspects of the programming, but helping people understand their relationship to it.
“Data literacy is about understanding and demystifying the process of what AI is doing, what datafication is doing,” says Wong. “The problem isn’t the technology itself; it’s the way it’s being distributed and rolled out to us. People are going to need to develop general digital literacy capacities in very short order, because concerns are arising about deepfakes, for example, misinformation and disinformation, because we have these social media tools that amplify information – wrong or right – in a very quick way.”
“What would be good is to have more organizations develop open-source language models,” adds Shwartz, “and more people in NLP working on completely different paradigms. Because we’re kind of stuck right now. In large language models, we have to patch all these problems of what's not working, like hallucinations or the fact that you need a lot of data so you can address representational bias.”
Somewhere at the intersection of public policy and individual responsibility, we will find a trade-off between ourselves as “products of data” and as “users of data.” For the past 20 years, smartphones and social media have rather sneakily created digital maps of our existence, and then used those maps to guide our choices in algorithmically selected directions. Ominous, yes, but also useful. Your phone tracked where you travelled, where you parked, and where you ate – but it also found you a faster route, a free parking spot, and a better taco place right on the way.
And this is where the leap to AI gets tricky: that taco place may or may not be there. It may be theoretical. It may be a hallucination. We don’t know. A mistake on a map used to be attributable to human error. With AI, we’re never sure. We’ve built this incredible machine to think for us, but because we can’t trust it, we have to become far more skilled at thinking for ourselves.
Despite future solutions adopted by policymakers to provide transparency into the data-gathering process, and by programmers to mitigate AI’s biases and constant surprises, ultimately one’s use of AI will come down to critical thinking. We need to understand the degree to which our activities have become commodified – not only to improve products and services, but also to generate what author Shoshana Zuboff, in her book The Age of Surveillance Capitalism, calls “behavioral surplus” – the data that helps companies predict the future behaviour of the user.
“I think the way we treat human beings as sources for data, and as commodities by extension (because that data is then bought and sold on the market), really flattens out the human experience,” says Wong. “It makes people seem like just economic actors. We have economic rights, certainly, but that’s just one small part of what it means to have human rights.”