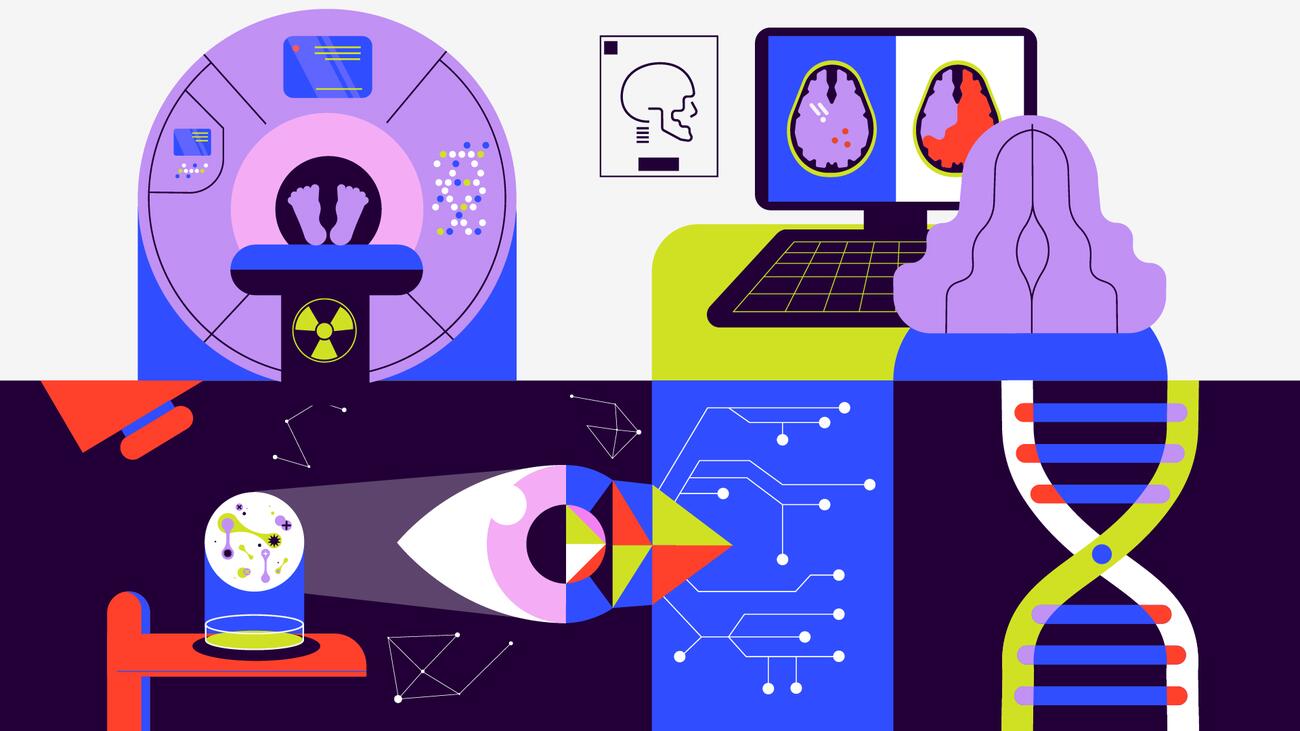
Illustration by Sanja Djordjevic on Unsplash
A healthcare revolution
Five UBC medical breakthroughs powered by AI.
Cancer survival predicted based on doctors’ notes
A team of researchers led by John-Jose Nunez, a psychiatrist and clinical research fellow with the UBC Mood Disorders Centre and BC Cancer, has developed an AI model that predicts cancer patient survival more accurately and with more readily available data than previous tools. It uses natural language processing to analyze oncologist notes from a patient’s initial consultation and picks up on unique cues – such as underlying health conditions, past substance use, and family histories – to predict the length of survival with greater than 80 per cent accuracy. “The AI essentially reads the consultation document similar to how a human would read it,” says Nunez, adding that such knowledge can optimize cancer care. “It might suggest health providers make an earlier referral to supportive services or offer a more aggressive treatment option upfront.” The model also has the advantage of being applicable to all types of cancers and can be quickly trained using local data to improve performance in specific regions.
Cancer cells analyzed using a smartphone app
A first-of-its-kind smartphone app is putting cell analytics directly into the hands of laboratory scientists. Named SnapCyte, the app produces accurate cell growth data faster than preceding technologies and at a fraction of the cost. “All you have to do is take the plate with the cell culture out of an incubator for a minute and put it under a microscope, where you can then snap a picture of it with your smartphone,” says Mads Daugaard, associate professor of urologic sciences at UBC and senior research scientist at the Vancouver Prostate Centre, where the app was developed. Used in the analysis of cancer cells and other diseases at a microscopic scale, the app aims to supercharge the development of novel, personalized medical treatments. Its AI algorithm is continuously learning and improving from the data received from users, says Daugaard, and the cloud-based platform means that the big data gathered can be easily shared to further advance research.
Sepsis detected in time to save lives
Sepsis is responsible for one in five deaths worldwide but is difficult to detect early on. It usually takes 24-48 hours before doctors can be certain of a case and treat it, but for every hour’s delay the risk of death increases by as much as 7.6 per cent. In 2021, researchers at UBC’s Hancock Lab pioneered a highly accurate method for detecting sepsis using machine learning. Arjun Baghela, a grad student under Dr. Bob Hancock at the time, led a large clinical genomics study of ER patients diagnosed with sepsis to gain insight into the dysfunctional immune responses it involves. The researchers used a machine learning model to identify sets of genes that can be used to predict whether or not a patient will acquire sepsis. Within one hour of a patient’s admission, doctors can now measure specific gene-expression biomarkers of sepsis and detect the specific dysfunctional response to infection that indicates development of sepsis, allowing them to quickly determine the most appropriate treatment.
Drug development supercharged by machine learning
Developing new drugs has typically involved a years-long process of screening millions of chemicals for their potential efficacy against disease. But during the COVID-19 pandemic, a UBC team led by Dr. Artem Cherkasov developed Deep Docking, a technology that has accelerated the process by up to 100 times. It uses machine learning to screen hundreds of billions of molecular structures to see how well they fit into a target protein in order to disable it – in the case of the COVID-19 virus, an enzyme crucial to its replication. The team was able to produce a short list of highly promising drug compounds for treating people infected with the virus in just 19 days, says Cherkasov, a professor in UBC’s department of urologic sciences. Beyond COVID-19, Deep Docking offers a blueprint for supercharging drug development in general, and the data collected is available to scientists around the world, giving them access to billions of molecules they can use for their research.
Rehab of stroke patients supported by “smart glove”
UBC electrical and computer engineering professor Peyman Servati, PhD student Arvin Tashakori, and their team at Vancouver startup Texavie have developed a groundbreaking “smart glove” capable of tracking even the smallest hand and finger movements and grasp forces of stroke survivors during rehabilitation exercises. The washable glove features a sophisticated network of highly sensitive sensor yarns woven into a comfortable stretchy fabric. The sensor data is wirelessly transmitted and analyzed using AI algorithms that allow care providers to fine-tune their exercise programs for the best possible results, even remotely, says Janice Eng, a professor of medicine who tested the glove with stroke patients. The glove is less expensive than motion-capture cameras but just as accurate, says Servati, whose team has developed advanced methods to manufacture it at a relatively low cost. The technology has numerous other potential applications, for example in augmented reality, robotics, and sign-language translation.