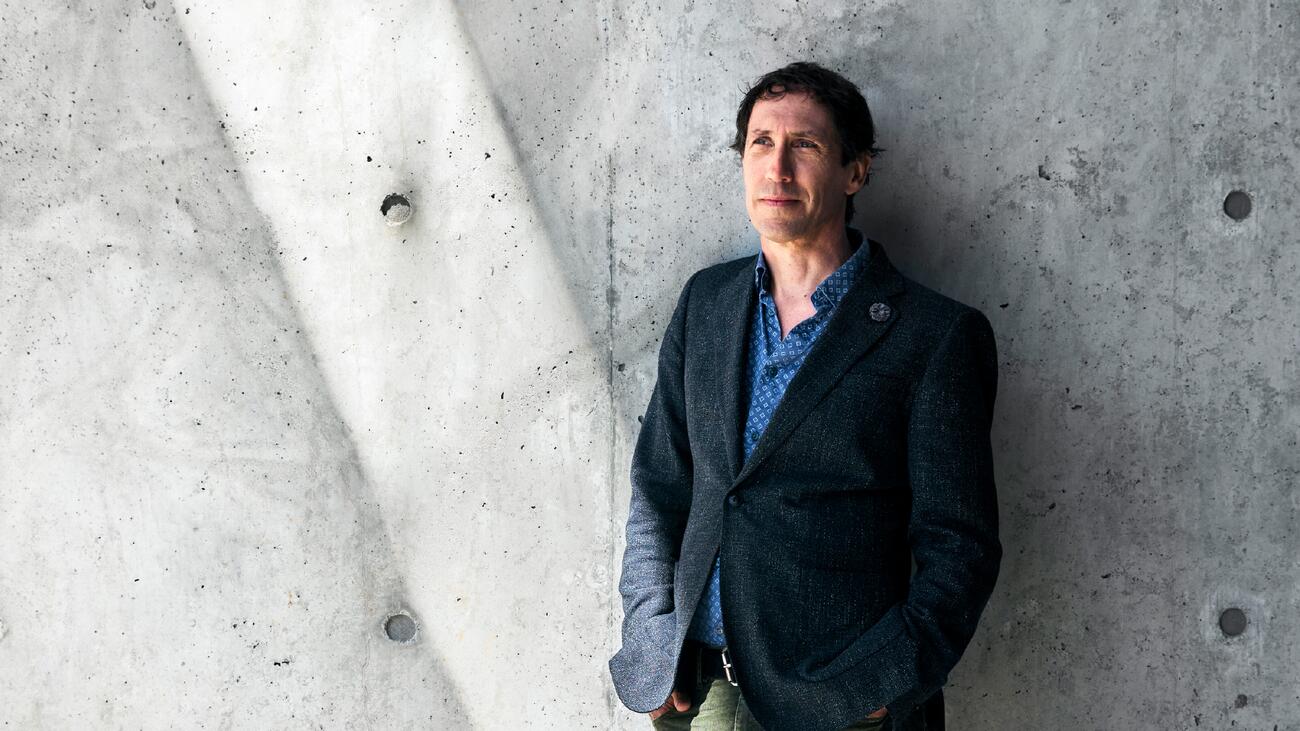
Photo by Kyrani Kanavaros
Can AI change life for the better?
A UBC prof is cautiously optimistic.
Kevin Leyton-Brown is an optimist about artificial intelligence (AI), but he doesn’t present himself as such. On the contrary, the professor of computer science and director of UBC’s Centre for AI Decision-making and Action (CAIDA) assumes a position that is deeply philosophical. When talking about the potential of AI as a force for good, he says, “You have to have humility. You have to avoid the colonialist impulse to say this is going to make life better.”
But when he starts talking about his own work advancing AI for social impact, for benefiting currently underserved populations, and even about the potential spillover effects of AI that has been developed for purely commercial purposes, Leyton-Brown keeps giving himself away. He’s pretty sure AI is going to make life better.
His philosophical urge seems to be hardwired. In the mid-1990s, finishing high school in the Toronto suburb of Richmond Hill after five years as one of those band kids (clarinet and guitar), Leyton-Brown says his first inclination was to study philosophy. But once at McMaster University, he cogitated on his prospects and switched his major to computer science, graduating with a BSc (philosophy minor) and enough academic acclaim to go to Stanford University, where he studied under computer scientist Yoav Shoham and collaborated with the Nobel Prize-winning economist Paul Milgrom.
Even there, Leyton-Brown maintained his philosophical bent. But instead of working directly on the meaning of life, he turned his attention to game theory, the mathematical study of how other people make meaning of life – or at least how they make strategy.
If you think of economics as a branch of math that tries to understand how people make decisions, the calculations are fairly straightforward if you’re only looking at one person or at people who all share the same interest. Game theory steps it up to looking at the dynamics of conflicting interests, which gets hard when there are two people, and harder still when there are more.
But, Leyton-Brown understates, “The internet facilitates a wide range of interactions that are larger and
more complex than traditional analysis can handle. My research extends game theory analysis to internet scale. It focuses on computational tools, auctions, and fast algorithms for solving hard problems.”
This is where you require the brute force – and, often, the colossal expense – of AI. As Leyton-Brown soon learned, you can get computers to beat chess grandmasters or to create large language models that write pretty credible essays. But it will cost you. A recent paper from Stanford University's Institute for Human-Centered Artificial Intelligence reports that, “OpenAI's GPT-4 used an estimated $78 million worth of compute to train, while Google's Gemini Ultra cost $191 million for compute.” Given that level of expense, Leyton-Brown says, “It’s clear that AI research is focusing on problems where there is money.” He also says, “It would be nice to put the same energy where there isn’t money.”
At least part of the time, that’s what he does, pointing out that it’s a privilege of being an academic: “You don’t have to work only on problems that are financially important for corporations.” In fact, he sees a moral obligation and an historical opportunity to leverage AI to benefit underserved communities, particularly in the developing world.
In addition to teaching a UBC graduate-level course about AI for social impact, Leyton-Brown has done a fair amount of hands-on work on what he calls “socially beneficial market design.” For example, during a sabbatical in Uganda, he noticed that a lot of subsistence farmers, and especially those in rural areas, were failing to sell their produce or having to accept a terrible price, while buyers elsewhere were overpaying or going wanting, and he thought, “We could do better.”
Working with a local partner, he developed Kudu, a mobile app that enables farmers to locate customers, and customers to find supply. Best of all, the app allowed everyone to agree on a benchmark price because it turned out that “people needed good price data more than they needed matchmaking.” When buyers and sellers both have better information, they both win.
The applications for this kind of AI-assisted innovation are innumerable. Leyton-Brown has also applied AI to improve peer-grading systems for large classes; to direct resources to charities such as food banks who need them most; and to automatically identify unexpected forms of bias in machine-learning systems, such as face recognizers. Graduate students in his AI for Social Impact class used AI to identify regions of Vancouver’s downtown core where drivers could save time, reduce traffic congestion, and lower greenhouse gas emissions by parking in city-owned off-street parking lots rather than in on-street metered spots, and presented their results to City Hall.
In one of his largest projects, Leyton-Brown designed the AI algorithm for a US Federal Communications Commission auction of broadcast radio spectrum that netted governments in the United States and Canada more than $7 billion. Governments north and south of the US border divided the broadcast spectrum up years ago, mostly giving it away to radio and television stations and then regulating the industry to ensure that signals didn’t overlap and create interference. But as mobility applications exploded in the last 30 years, there’s been increasing demand for that virtual space. Government tried placing new users in higher frequencies, but even there they started running out of room. And besides, high-frequency signals can’t pass through buildings. To penetrate concrete, you need the long-wave, low frequencies.
Reorganizing this scenario was complicated and potentially super expensive. Even though many television stations were hardly using their spectrum (because most of their clients had moved to cable), they didn’t want to just give it up. So, government set about trying to buy back and redistribute all of that virtual space – doing a simultaneous deal with 2,000 television stations in every heavily overlapping jurisdiction north of the US-Mexico border. They could never have managed the incredibly complicated sale without the enormous AI assist.
As AI finds more applications, society as a whole will become richer. It’s a question of how we want to distribute the gains.
– Kevin Leyton-Brown
The takeaway from all this, Leyton-Brown says, is that AI can bring incredible benefits, not always because people are trying to use it for good, but because “it’s basically free labour.” And as AI finds more applications, “living standards will rise; it’s a question of how we want to distribute the gains.”
Leyton-Brown also says he isn’t worried about big AI innovators hoarding the benefit. He says, “AI is expensive to make, but cheap to deploy.” As the viral distribution of ChatGPT demonstrated, “it’s hard for these things to be bottled up or controlled by a small number of people.” And once a new tech is loosed in the wild, “you can’t limit its access or what it gets used for. As a big complicated thing that we all get a piece of, AI is a kind of democratizing force.”
The question now is where else can AI have the best effect, for social as well as commercial advantage? Leyton-Brown looks to what he sees as an obvious area of application: mitigating climate change, a global and hugely complicated problem that nevertheless has findable solutions. Most especially, he says, we should be able to figure a way to “reduce carbon output without tanking the economy.”
There are also areas that are less predictable but – to Leyton-Brown, at least – obvious once you bring them up. For example, there is promising research using AI to analyse electrocardiograms to seek out heart and circulatory issues that even the best radiologists can’t identify as effectively. Leyton-Brown looks at it as a pretty direct application of machine learning, where you can train a computer with hundreds of thousands of previous scans and ask it to identify patterns.
And if those kinds of innovations wind up displacing workers, Leyton-Brown also thinks that could be a win. Just like how the introduction of ATMs actually led banks to increase the number of in-person branches by refocusing on customer service and selling financial products, radiologists can turn their attention to more high-value tasks where AI still can’t compete.
Still philosophical, he’s not promising the transition will be easy – “No one I know thinks these questions are resolved” – but, he says, “if society is richer because we are more productive, it will be up to us to decide how best to use, and distribute, the money.”
If you’re looking to anticipate AI disruption, trying to decide where to find jobs AI can’t displace, Leyton-Brown suggests the kinds of roles where human intervention is most desirable: teachers, massage therapists, coaches, or family doctors. And, despite recent reports that “computer scientist” is the next job that AI is about to render obsolete, Leyton-Brown says it’s quite the opposite. AI might be able to write elementary code, but “like most knowledge work, computer science requires a lot of creativity, much of it working in teams that requires rich people skills, and hence isn’t the sort of thing that can easily be replaced by AI.”
That broad understanding – and especially Leyton-Brown’s consciousness of the importance of teamwork – is reflected in the way that UBC has established its own AI concentration: not in a stand-alone school, but deeply integrated across the institution. As UBC’s main AI research organization, CAIDA includes more than 100 professors and their research associates, spanning 27 departments, schools, and institutes. The centre focuses on the development, analysis, and application of AI systems for decision-making and action, enabled by core AI technologies such as machine learning and automated reasoning.
Within CAIDA, Leyton-Brown also directs AI Methods for Scientific Impact (AIM-SI), a cluster of faculty dedicated to increasing UBC’s teaching and research capacity. Seeing a demand for AI teaching and research from across all scientific disciplines, and from outside the sciences as well, AIM-SI is distributing its effort in a way it hopes will lead to a broader assortment of breakthroughs, even as it produces a larger pool of highly-qualified graduates for Vancouver’s tech sector.
So, UBC is not trying to build an enclosed AI hothouse, but rather to develop capacity across the institution to understand, manage, and innovate with AI and to generate a diverse group of scholars and graduates who can support that effort, within the university and in a wider community – pursuing a vision in which AI helps to make everyone’s life better.